Libra introduces a groundbreaking paradigm in the AI agent landscape - the Vibe Agent.
Cutting-Edge Technology
1. Low-bit Quantization Technology
Libra employs mixed-precision quantization and Reasoning-Aware low-bit representation calibration techniques to precisely compress cutting-edge large models (QwQ 32B, DeepSeek-R1-70B, Deepseek R1 671B, etc.) into 3/4-bit mixed-precision representations compatible with Apple's consumer-grade ARM-based computing architecture, seamlessly integrating with the Apple MLX machine learning inference framework. In terms of performance preservation, the performance loss of conventional large language models is lower than 1%, while memory requirements are significantly reduced by 75% and more compared to FP16 mode.
Libra's technology stack successfully overcomes the precision bottleneck of traditional quantization methods through a carefully designed mixed-precision representation coupled with recalibration strategies, perfectly protecting the "Super Weights" that influence the model's core capabilities while meeting the requirements to run on consumer-grade hardware.
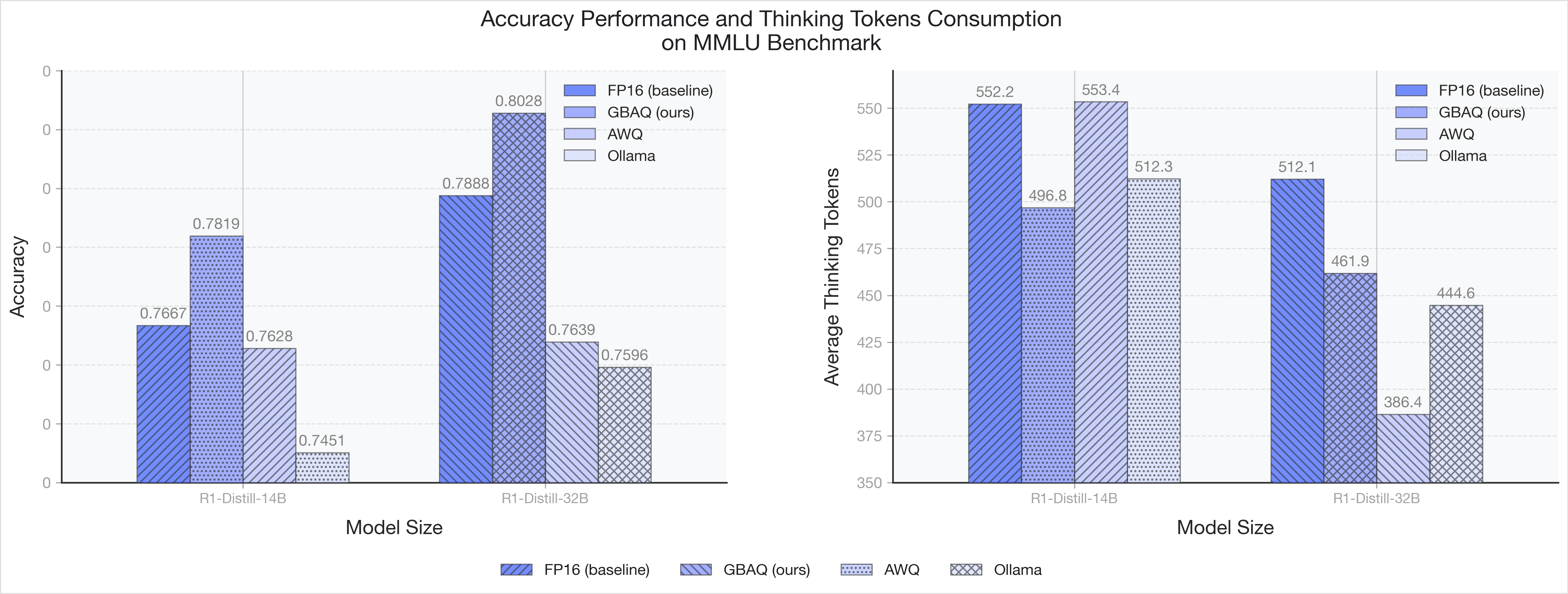
2. Adaptive Context Management Architecture
To overcome local device resource limitations and model Context window constraints while achieving effective token aggregation, Libra employs an event-driven Token Vibe Orchestration (TVO) strategy. TVO employs a JSX-based hierarchical resource scheduling strategy to efficiently integrate frontend, backend, and historical interaction data, and uses dedicated models to perform speculative summarization and priority prediction of the original context, enabling the model to anticipate user interaction intent and rearrange the most relevant context fragments, thereby achieving exceptional context understanding capabilities in environments with limited computational resources.

TVO architecture can prioritize the retention of high-value information, significantly improving model response quality.
3. Responsive Orchestration Engine
Libra proposes an innovative Meta Agent-Orchestration (MAO) framework for resource scheduling of Vibe Agents generation. The MAO framework customizes dedicated policy agents for Orchestration scenarios, internalizing complex Orchestration-related knowledge, enabling the system to autonomously reason and predict optimal collaboration paths. Based on efficient database strategies, MAO can systematically integrate numerous external tool chains and provide real-time front-end and back-end interaction context. This design ensures seamless collaboration between all components while maintaining efficient operation even with limited local device resources. As an important supplement to the framework, MAO also constructs dedicated predictors for data flow layer availability, achieving usability verification of natural language generated agents through real-time graph connectivity verification, effectively minimizing the risk of task failure.
